Question the hype: why 80% of AI projects fail
and how leaders can help
Featured UQ Business School expert Dr Evan Shellshear with industry expert Doug Gray
Research reveals a staggering failure rate for new data science and AI projects. For the best chance of success, business leaders should counter the hype surrounding AI with a healthy dose of realism to employ it in meaningful ways.
Artificial Intelligence (AI) is embedded in our daily lives, from the spam filters that monitor our inbox to the new music suggestions Spotify knows we’ll love. The technology is enchanting, powerful and persuasive. Yet, its growing pervasiveness also strikes fear and mistrust in people and organisations.
The hype surrounding Analytics, Data Science and AI (ADSAI) can influence an organisation’s investments in its tools and technologies. But the same hype can polarise public opinion – from doomsday predictions of 300 million job losses to utopian visions of free healthcare, economic stability, and social equality.
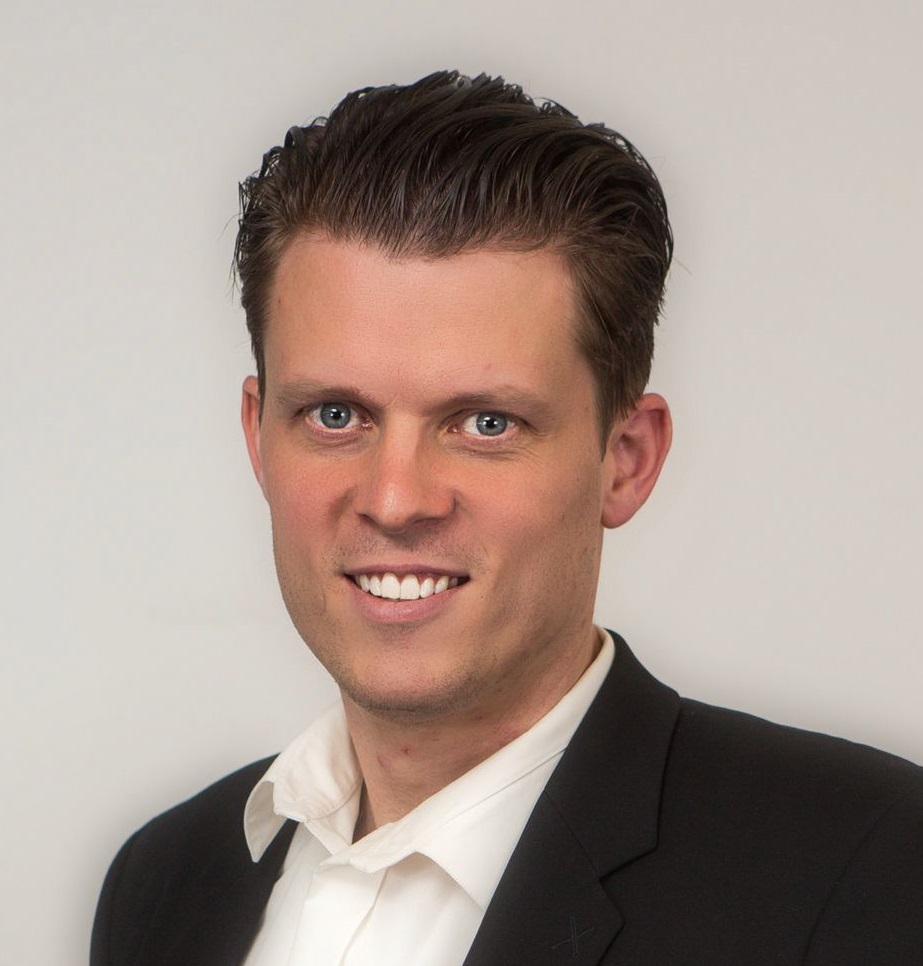
The reality, however, tells a different story – uncovered by research and industry case studies from UQ Business School expert Dr Evan Shellshear and his industry colleague Doug Gray in their book, Why Data Science Projects Fail: The Harsh Realities of Implementing AI and Analytics, without the Hype.
Through academic investigation and industry insight, they found that for every AI breakthrough and brilliant new application, a staggering number of projects fail before meaningful launch, costing organisations billions in lost resources and revenue.
“Eighty per cent of AI projects fail,” Dr Shellshear summarised.
“Just to be clear, that’s not the AI projects you read about, like the Uber self-driving vehicle that hit a pedestrian or Microsoft’s Twitter Tay chatbot. These are projects that fail to get off the ground at an organisational level before the public ever knows about them.”
The top reasons for failure – and how to overcome them
While combing through academic literature, peer-reviewed articles, blog posts and podcasts, the duo found patterns and commonalities among the failures. They discovered most failures had nothing to do with the data science not performing well and everything to do with issues of strategy, process, people, and ancillary technologies.
“The top reasons were factors like choosing to ‘fix’ a problem that’s not aligned with the business strategy, not having access to the right resources, the data being in the wrong places or in silos, not having high data quality and underestimating how difficult these projects are to take from modelling to implementation,” Dr Shellshear said.
“Ultimately, the 2 biggest causes of failure were neglecting to build a need in the organisation for the project and a lack of quality processes to have the right data in the right place at the right quality.”
Crucially, the authors discovered that many of the catalysts for failure could be fixed by most business leaders across an organisation – even those without deep AI knowledge.
5 tips to avoid common failures
- Understand the real business problem: Identify strategically important projects in an organisation where AI and data analytics can help fix a genuine business problem.
- Audit the data: Find out where the data is, whether it’s good, and if additional data collection is needed.
- Focus on communication and change management: As ADSAI can seem threatening to many people because it brings significant disruption, it’s essential to dedicate time and resources to help your team embrace change.
- Set realistic expectations: Start small and simple, and acknowledge that, like all new technology, the project may experience setbacks and require creative solutions.
- Assess the company culture: ADSAI projects need the support of senior leaders, so invest time in ensuring your leaders are analytically mature, informed and project advocates.
Case study: solving a problem that isn’t a business priority
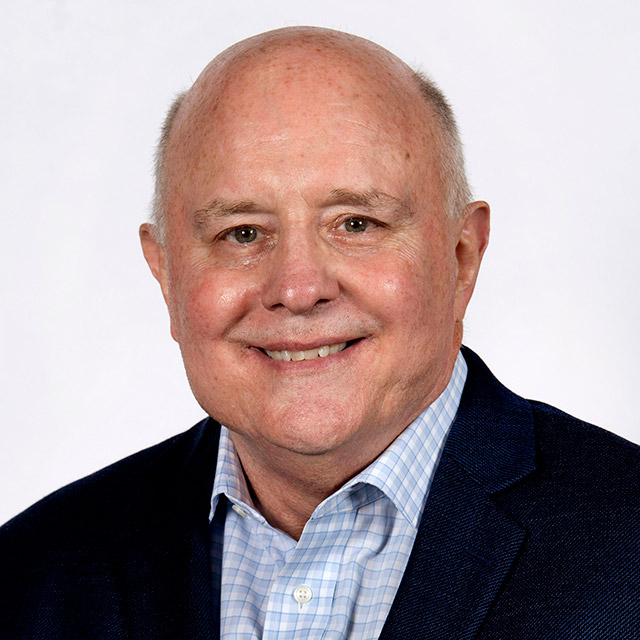
Mr Gray is an Adjunct Professor in Business Analytics, Data Science and AI at Southern Methodist University in Texas and a data scientist with decades of experience in the travel sector, including at American Airlines, Southwest Airlines and Expedia precursor Travelocity. He told the story of an airline that turned to AI to speed up boarding times at departure gates.
“The data science team used video cameras in the jet bridge, coupled with a computer vision algorithm, to scan carry-on baggage and estimate each bag’s volume to predict in real-time when the plane cabin’s overhead bin space capacity would fill up,” Mr Gray said.
“Typically, cabin-stationed flight attendants would monitor the boarding process to guesstimate when the overhead bins would fill up and then notify the boarding door stationed flight attendants to start gate-checking the remaining bags.
“The issue in this case study isn’t with the computer vision algorithm technology or its application. The model worked, but it had no appreciable impact on the boarding time.
“So, what did they accomplish? They proved that computer vision could work in this environment. They spent $1 million and got no tangible value from it. Boarding times remained about the same.”
RESULT: The airline shelved its new AI tool as the results didn’t warrant the hefty cost.
“Excessive focus on a model, technique or technology because it’s cool or interesting without consideration of the true potential business value and economic or operational impact can waste valuable time and resources, something few companies can afford,” Mr Gray added.
Read more: What will a robot make of your résumé? The bias problem with using AI in job recruitment
Learning from failure
Dr Shellshear said their research into ADSAI failure drew from a landmark 2013 US study into how people learn.
“Individuals learn more from their successes than their failures,” he said.
“But when we look at others, we learn more from their failures than their successes. There might be a little jealousy, a little envy, or maybe we like to be critical, but whatever the reason, you’re going to learn the most from others’ failure rather than thinking, ‘Aren’t all these people great?’.”
Failure offers feedback and is a crucial element of any great discovery.
“Most of my projects were all hard fought. They all failed more than once along the way and then had to be recouped and re-reconstituted,” Mr Gray said.
Dr Shellshear emphasised the importance of innovation for AI.
“AI needs the ability to take a risk and fail,” Dr Shellshear said.
“AI needs the ability to discover as you’re going. It’s not a given that the project or tool will work. It’s highly likely it will fail, and that’s not always a bad thing.”
Case study: when there’s no human buy-in
Finance is one industry hyping up AI to decrease risk and increase returns. The authors recounted what happened when a financial institution engaged an external consultant to build an AI tool to predict defaults on home loans. Initial testing of the machine-learning model showed it would improve the company’s bottom line. But 2 main issues caused serious problems.
Issue 1: The company already had an actuarial team that wasn’t actively involved in the project. This team had built for-purpose functioning statistical models that they understood and trusted and had already incorporated into their systems.
“From day one, the actuarial team was suspicious of the machine learning approach and didn’t understand why a replacement was necessary,” Dr Shellshear noted.
Issue 2: The external data scientists developed a plan without consulting the company’s IT team first. Throughout the project, IT followed its familiar and proven security protocols, inadvertently slowing down the external team’s access to environments, data and the cloud environments.
RESULT: The situation became untenable for the consultants and deteriorated when the original project champions – the CIO and CEO – left the company.
“As the dominoes began to fall, the data science team lost the support of the rest of the company. In particular, the actuaries had zero interest in the new approach replacing their existing models,” Dr Shellshear said.
“From here, the opportunity to increase profitability and give customers a better experience ultimately died with a final whimper as the company went back to business as usual … to the quiet satisfaction of the actuarial staff.”
Read more: Is your team wary of new technologies? Explore how to build workplace trust in AI
Set for success –
the final word for business decision makers
#1. Forget the hype
“Slow down and avoid the hype. It sounds paradoxical because it’s so fast-moving, but you need to slow down, look at your business strategy and avoid the hype,” Dr Shellshear said.
#2. Don’t put the cart before the horse
“Don’t become so enamoured by ADSAI tools and technology that you run around the building until you find a problem to work on,” Mr Gray said.
“You want the problem to come to you. Start with the problem and then pick the solution. Otherwise, there’s a big risk that you’ll burn up a lot of financial and political capital if you make too many mistakes.”
#3. Focus on the finances
An AI system should be adopted by an organisation if, and only if, the profit obtained is greater than the opportunity cost of that project. Opportunity cost is the value of the next best alternative – be that a legacy system or an alternative solution.
#4. Champion your cause
Companies that hastily launch AI development without a clear definition or cause can unwittingly leave leadership teams behind. Business decision makers who don’t understand the need, process and reasons for ASDAI projects don’t have 100% buy-in, so they won’t offer 100% support.
Set leaders up to champion the project by setting business targets with clear commercial goals, defining success and communicating the project’s journey – including its setbacks and failures.
“AI is like many powerful technologies that have come before it,” Dr Shellshear said.
“It has incredible transformative potential – either to drive meaningful innovation and efficiency, or, if misapplied, to drain resources chasing solutions in search of a problem.
“The key is to stay grounded, stay strategic, and never confuse a tool with a silver bullet.
Learn how UQ Business School Trust, Ethics and Governance Alliance researchers are enhancing our understanding of public trust in AI
Dr Evan Shellshear
Dr Evan Shellshear is an Adjunct Professor at UQ Business School. He’s an expert in industry transforming technologies and methodologies, from software to consulting with data, analytics and AI.
Doug Gray
Doug Gray is an Adjunct Professor in Business Analytics, Data Science and AI at Southern Methodist University in Texas and a data scientist with decades of experience in the travel sector, including at American Airlines, Southwest Airlines and Expedia precursor Travelocity. He’s currently Director, Data Science, End to End Fulfillment at Walmart Global Tech at Walmart’s home office in Arkansas.